A credit engine is a sophisticated software system that assesses and analyzes creditworthiness, providing lenders with valuable insights to make informed decisions. By evaluating factors such as credit history and financial behavior, credit engines help streamline the loan approval process, enhancing efficiency and reducing risks. This technology plays a pivotal role in modern financial ecosystems, optimizing lending practices.
Reimagine Your Order-To-Cash with AI
Touchless Receivables. Frictionless Payments.
Credit Risk
Receivables
Collections
Deductions
Cash Application
Customer EIPP
Bringing the Trifecta Power - Automation, Analytics, AI
Recommended Digital Assets for You
Suggested Resources
Digital World-class Leaders Use Emagia Order-to-Cash
Case study
Case study

Case study
Cali Bamboo Boosts Digital Credit Decisions with Emagia
Case study
Digital Credit Transformation for American Heart Association
Add AI to Your Order-to-Cash Process
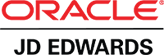
AR Automation for JD EDwards
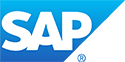
AR Automation for SAP
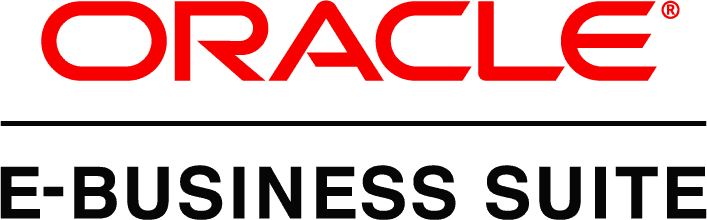
AR Automation for Oracle
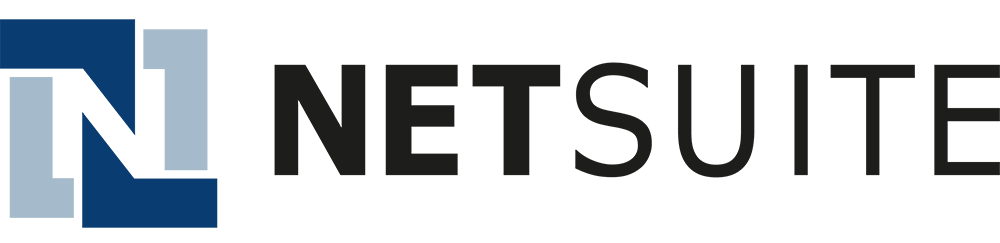
AR Automation for NetSuite
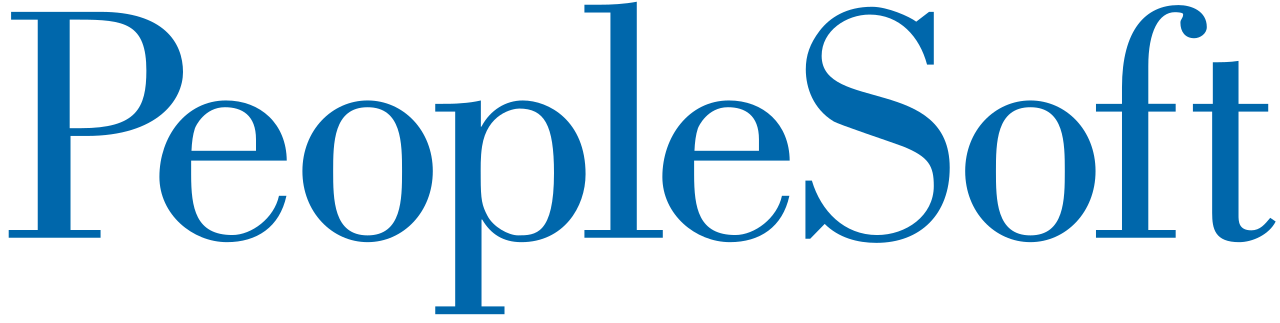
AR Automation for PeopleSoft
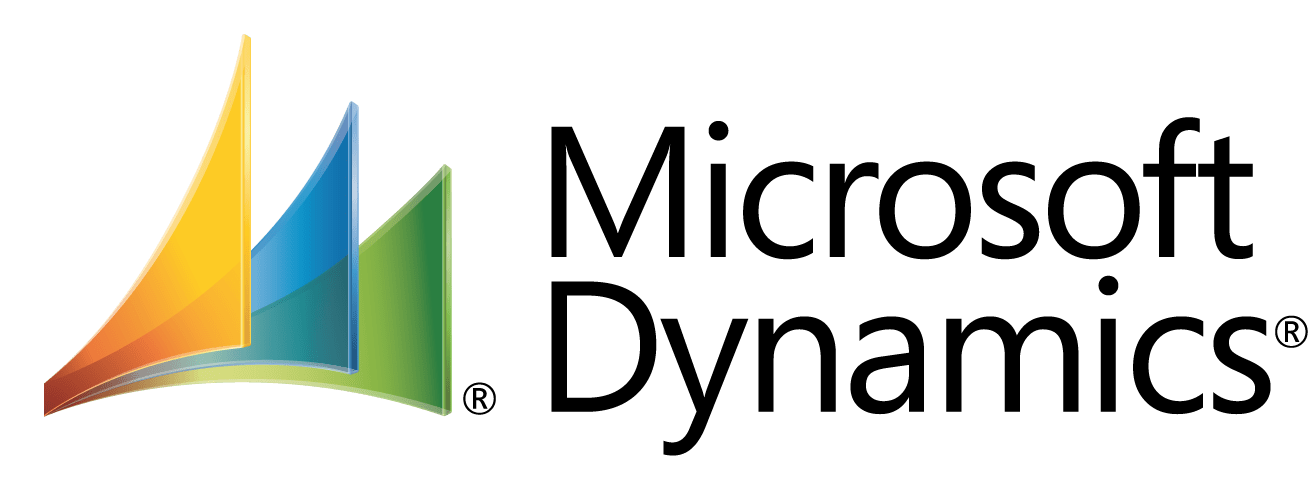
AR Automation for MS Dynamics
Emagia is a leading provider of AI-powered Order-to-Cash (O2C) automation platform that modernizes finance operations for midsize to large global businesses. Many global businesses and shared service centers use Emagia’s Enterprise Receivables Management System to transform to digital world-class operations in credit, invoicing and payments, receivables, collections, deductions, cash application and cash forecasting. Emagia solutions improve their customers DSO, cash flow, credit risk, operational cost, compliance and profitability.